Safeguarding Patient Privacy in AI Development: Insights from Dementias Platform UK
24 April 2024

Understanding AI model risks is imperative to allowing the safe development and sharing of AI models which protect patient privacy while still enabling open and reproducible science. Over the past few months, our team at the Dementias Platform UK (DPUK) Data Portal has been leading work, funded by DARE UK, to evaluate AI model risks in sensitive healthcare data. This work aimed to gather valuable perspectives from members of the public, AI researchers, and data providers to understand concerns of AI development and release from Trusted Research Environments (TREs).
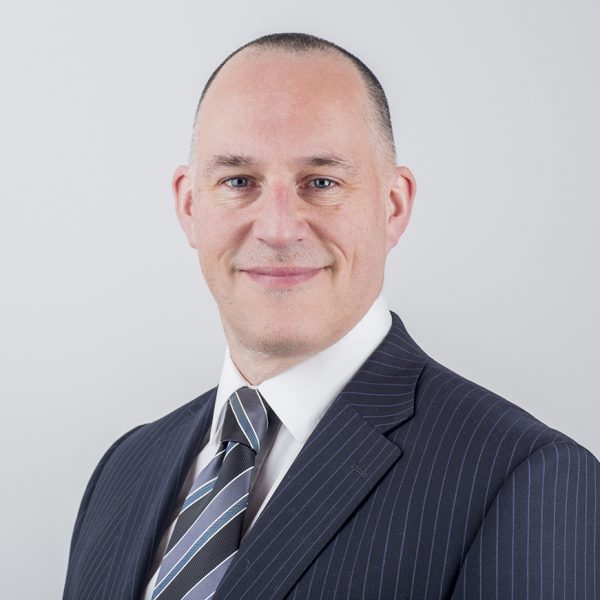
Professor Simon Thompson
Professor of Health Informatics, Swansea University
“With the rapidly evolving landscape in the development of AI models on sensitive healthcare data, it has never been more important to consider the risks that these models pose to patient privacy and what role Trusted Research Environments (TRE’s) have in ensuring the responsible development of these models and the safe release of them.“
Workshop highlights
Public Workshop
From our first workshop, we found that members of the public overwhelmingly preferred TREs to be used for developing AI models on their health data, but stressed the importance of public involvement at the decision stage to ensure models are being developed and shared in the public benefit. Following this, DPUK aims to incorporate public involvement at the decision stage process to ensure that AI models are being developed within the public benefit. To improve our decision process, researchers will have to provide additional documents in their application such as a lay summary for members of the public, and an AI risk impact assessment detailing how they plan to mitigate concerns.
Research Workshop
From the researcher workshop, it was clear that most researchers aren’t aware of the risks their AI models pose and how to appropriately mitigate these for safe release and deployment into the real world. Therefore, training and resources are crucial to enable AI models to be developed responsibly in health data. Researchers also felt the need for tools to help generate and evaluate safe data for training AI models. This is why DPUK has developed tools for generating and evaluating synthetic data for developing private models, and has started developing courses for researchers to learn about AI risks and how they can be appropriately mitigated depending on the types of data and models used.
Data Owners Workshop
Furthermore, data owners felt that they lacked the expertise to comfortably assess AI model projects and relied on the TRE to guide them to help make decisions therefore, they expressed the need to quantify risk of releasing AI models in various scenarios. Additionally, it was clear that data owners felt that running attack simulations on AI models provided adequate assurance that they could be released from a TRE. From this, DPUK has developed the AI Risk Index to quantify the risks of AI model release, taking into consideration a range of different data types, AI model types, sharing scenarios, and vulnerabilities. We also plan to use the SACRO AI-SDC tool for running attacks on AI models to evaluate robustness against attacks
From the results of these workshops, we put together a series of recommendations, tools and materials for assessing AI models being released from a TRE. However, it was decided that the need for releasing AI models from a TRE should be reduced as much as possible, without hindering scientific research.
Recommendations
There are three main reasons why a researcher may want to export an AI model:
- to publish on a platform such as GitHub for open and reproducibility reasons
- to further train the model on external datasets
- to deploy into clinical practice.
In all three cases, the model doesn’t necessarily need to leave the TRE.
In cases where (1) the researcher wants to publish an AI model, they should be published via the TRE where researchers can apply and access that AI model in the same way that derived data is. This allows the AI model to stay secure within the TRE while allowing access for reproducibility and validation. DPUK plans to implement infrastructure and tools to enable sharing and access of AI models developed on the portal to other researchers to enable open and reproducible science.

Lewis Hotchkiss
Research Officer, DPUK
“Open and reproducible science has long been an issue for AI research, and the privacy risks posed to releasing these models play a big role in this. Therefore, a key recommendation from this report, is the utilisation of the FAIR framework for AI models to ensure that they can be accessed in a secure manner, while still enabling open and reproducible science By hosting AI models through TREs, rather than openly accessible platforms such as GitHub, we can enable AI to be FAIR, while also fulfilling our duty to protect the data that was used to train those models. Overall, this work further showcases the role of TREs and the importance of them to protect data while enabling beneficial AI research to take place.“
When external data is needed for training or validation (2), this can be achieved through DPUK’s secure federation solutions to enable access and training on other datasets.
If an AI model is ready to be deployed into the real-world (3), then secure hosting offers the most suitable solution, where the model can stay within the portal, and the TRE offers ways to securely query it and receive predictions externally. This enables the safe translation of AI models into clinical practice while ensuring utility isn’t affected. We are actively working with projects to find suitable solutions to be able to query AI models from within the TRE.
However, if the researcher still requires an AI model to be released outside of the TRE, then privacy-preserving techniques should be implemented by the researcher and will be rigorously evaluated by the TRE to ensure that it is safe for release.
These perspectives, recommendations and materials help TREs move forward to enable important AI research to take place while still protecting the data they have been entrusted with.